In today’s digital landscape, data is crucial for business success, driving insights, decision-making, and AI advancements. As companies handle large volumes of data, ensuring its quality is vital. Poor data not only hampers growth but also poses compliance risks. With AI systems like ChatGPT and Copilot relying on clean data for accurate predictions, the importance of reliable data is heightened.
The saying “garbage in, garbage out” has never been more relevant; if the data is flawed, the insights and outcomes derived from AI will be equally compromised.
If the data is flawed, the insights and outcomes derived from AI will be equally compromised.
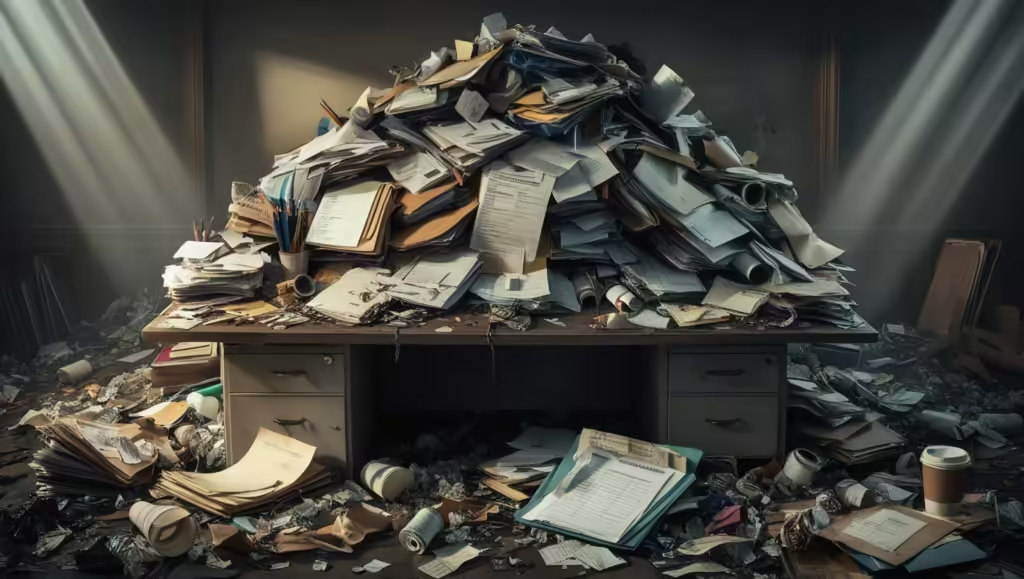
Defining and managing data quality
Although the term “data quality” is commonly used, people’s views on data quality can be very different, even when discussing the same set of data used for the same purpose. There is often a lack of common understanding among key stakeholders about what data quality really means.
Data quality can be defined as a measurement of qualitative and quantitative conditions that determine whether the data is fit for its intended use in a business process or operation.
The quality of data can be evaluated against defined dimensions (i.e. accuracy, completeness, conformity to standards, consistency, coverage, timeliness, uniqueness…) as well as against the business processes associated with its production. The more relevant, available, complete and accurate the information, the better chance profitable business insights will be created.
AI’s Popularity Surge
AI has captured the spotlight for the general public, with weekly global Google searches underscoring this trend:
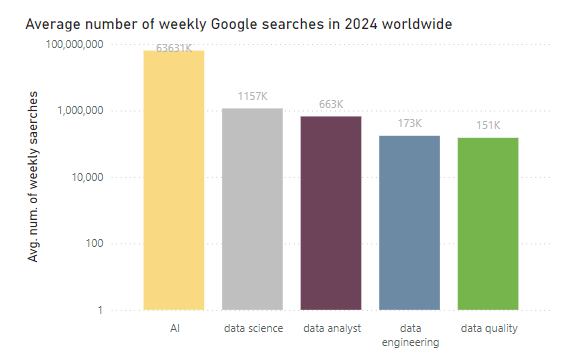
Search interest in AI is about in the order of 100 times greater than in core data principles like data quality and data engineering, while topics like data science and data analysis show roughly 10 times the interest. This stark contrast in popularity became (even more) pronounced with the release of ChatGPT in November 2022, which catalyzed a global surge in AI curiosity.
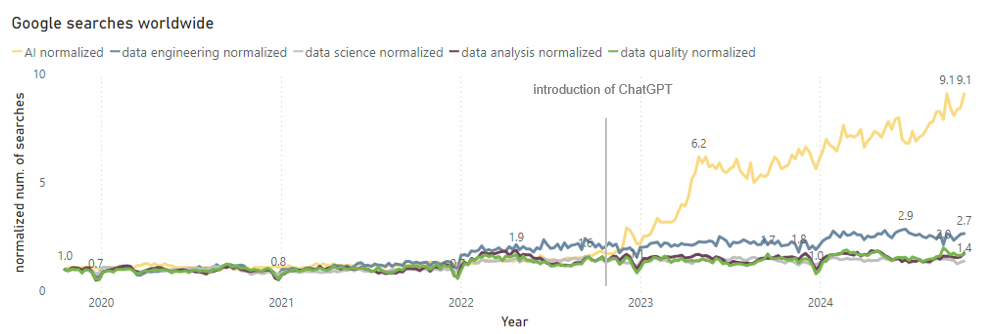
From 2019 to 2022, Google searches for AI nearly doubled. Since ChatGPT’s release, this interest has skyrocketed to roughly nine times its 2019 levels, marking a clear shift in consumer focus. In contrast, core data principles like data quality and data engineering have grown more steadily over the past five years, with search interest increasing by 1.5 to 2.5 times
Today, as AI gains widespread attention, consumers expect more advanced and integrated data solutions from companies. And here, data quality plays a key role.
Data quality: The key to (data) success
As AI models become more accessible and widely available, the real source of competitive advantage shifts to the quality of the data that fuels them. Even the most advanced AI systems will fall short if the underlying data is poor, ultimately diminishing the potential benefits that AI can provide.
As AI models become more accessible and widely available, the real source of competitive advantage shifts to the quality of the data that fuels them.
To tackle data quality challenges effectively, businesses should follow a three-step approach:
- Prioritize high-value data: In a sea of data, it’s impractical to address everything at once. Start by identifying and improving the data that directly impacts your most critical AI and business use cases. This focused strategy not only maximizes return on investment (ROI) but also ensures the accuracy and reliability of key data, providing a solid foundation for AI initiatives.
- Scale incrementally and hire the right talent: After optimizing high-value data, gradually expand your data quality efforts. Scaling effectively requires the right expertise—hiring skilled data professionals is crucial to managing this process. Experienced data engineers and data scientists can not only assess the quality of your data but also implement the right tools and methodologies to maintain it. By bringing in the right talent, businesses can ensure that their data quality efforts evolve in alignment with regulatory demands and business needs.
- Establish a strong data quality framework and culture: As AI capabilities advance, robust data governance becomes essential. Implement frameworks that promote ongoing data quality enhancements, while also ensuring compliance with privacy and security regulations. Building a culture that values data quality, supported by the right talent, is crucial to sustaining long-term success.
Remember, improving data quality is not a one-off task—it’s a cyclical process that requires commitment, strategic focus, and the right people to drive it forward. This step-by-step approach allows for continuous refinement, preventing overwhelm and allowing your team to build on small, sustainable wins.